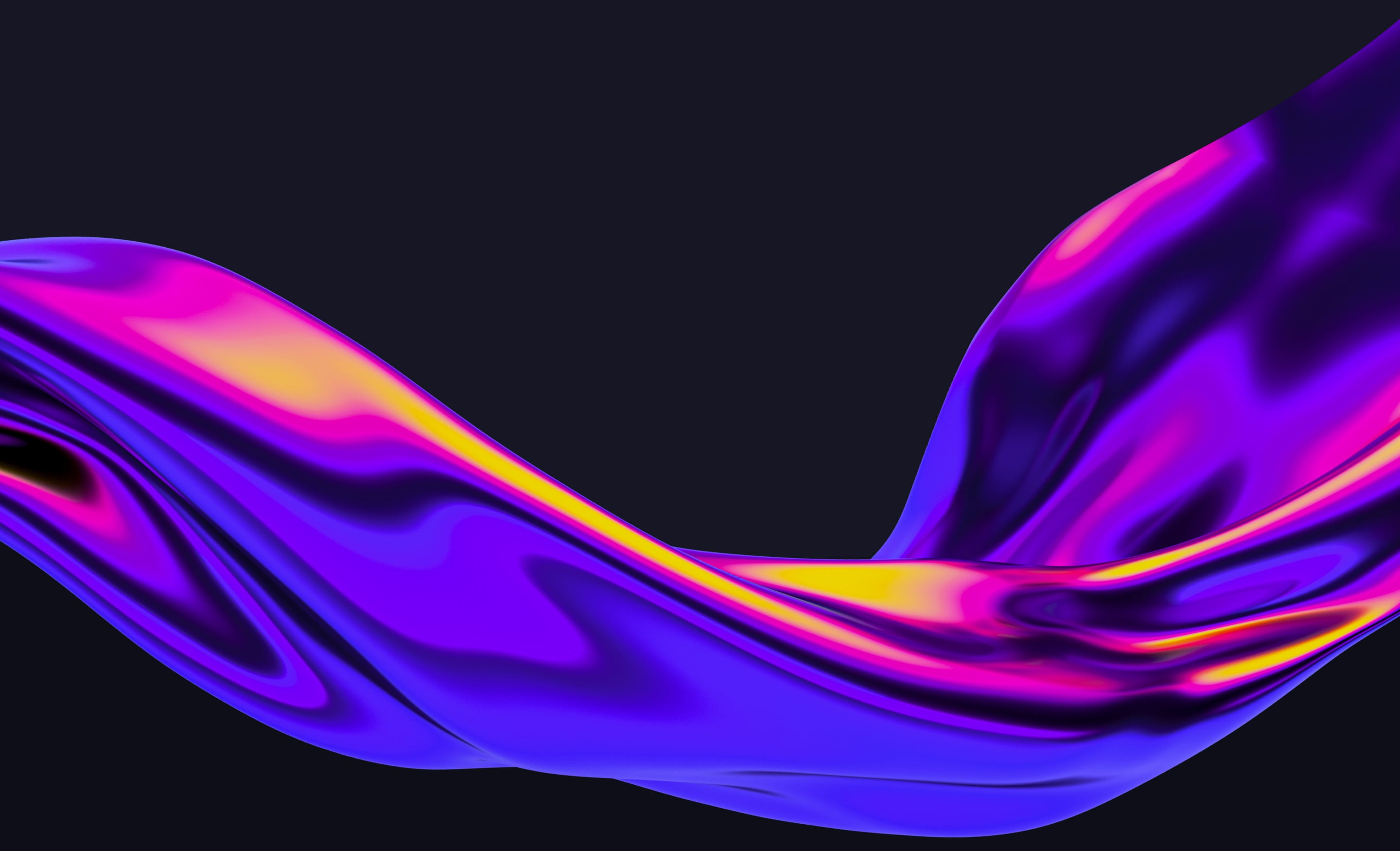
Most enterprise machine learning applications today are based on supervised learning and as such depend on costly and time-consuming manual data labeling efforts. Yet as much as we invest in data labeling, most ML practitioners realize that our “ground truth” is often anything but. As organizations invest in modernizing their ML stack, the integration between MLOps and data needs careful consideration.
In this talk, we discuss the main challenges that organizations face when dealing with labeling, how these problems are currently being solved, and how techniques like active learning and weak supervision could be used to more effectively create training data. We review where and how these techniques fit into the model development workflow, and how they support and are supported by MLOps efforts.