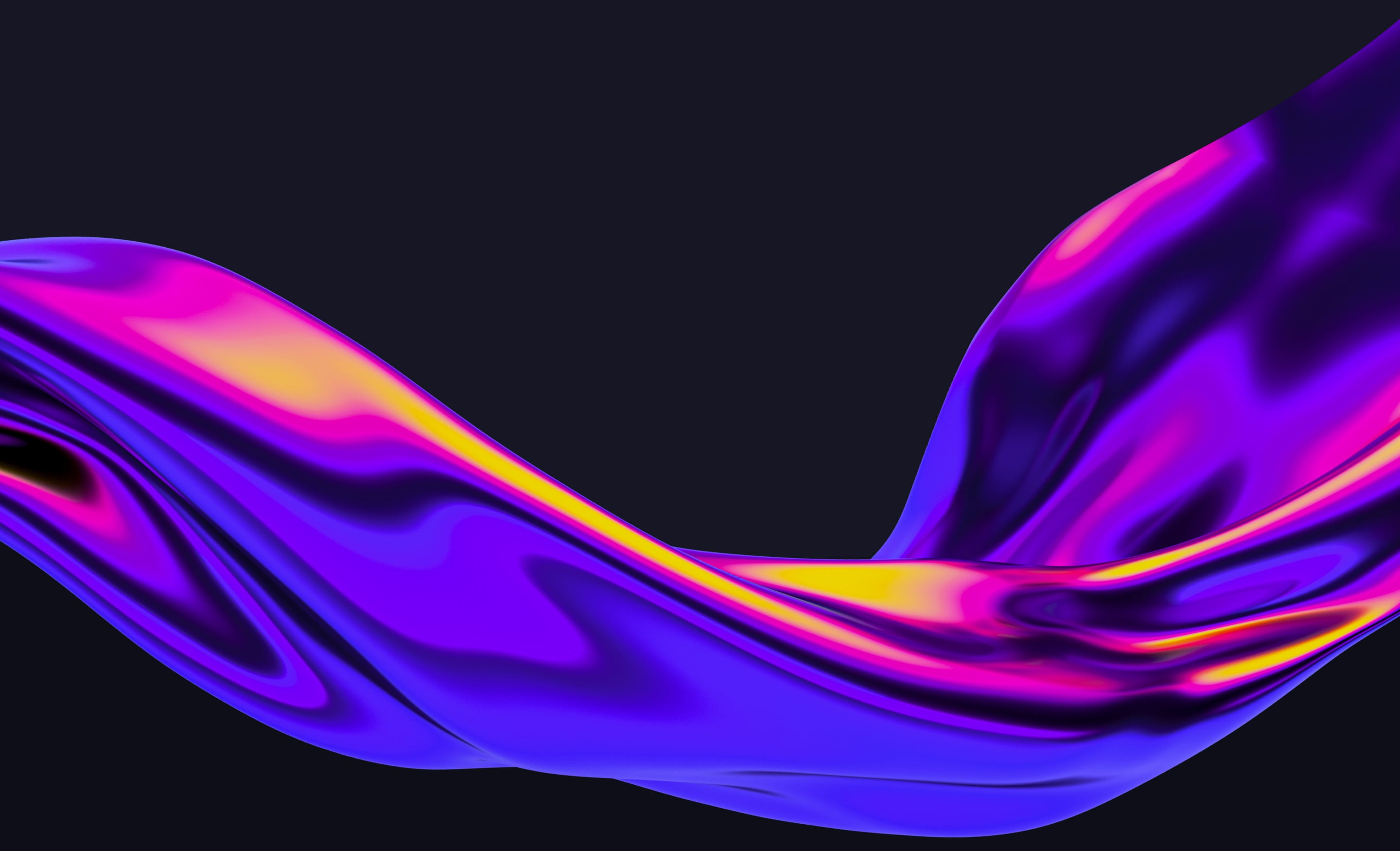
Dive into the latest episode of "Grounded Truth" hosted by John Singleton as he discusses "Retrieval Augmented Generation (RAG) versus Fine Tuning in LLM Workflows" with Emmanuel Turlay, Founder & CEO Sematic and Airtrain.ai and Shayan Mohanty, Co-founder & CEO of Watchful.
Links:
- Podcast: YouTube: https://youtu.be/pdQDO3UTjVE
- Spotify: https://open.spotify.com/episode/6TKHhvDuWtKKCyf7xUcOLk?si=2e05d9044fdc4b2e
- Apple Podcast: https://podcasts.apple.com/us/podcast/grounded-truth/id1675140111?i=1000638136227
Visit http://Airtrain.ai on YouTube: https://www.youtube.com/@AirtrainAI
🤖 RAG: Retrieval Augmented Generation
- RAG involves putting content inside the prompt/context window to make models aware of recent events, private information, or company documents.
- The process includes retrieving the most relevant information from sources like Bing, Google, or internal databases, feeding it into the model's context window, and generating user-specific responses.
- Ideal for ensuring factual answers by extracting data from a specified context.
⚙️ Fine Tuning
- Fine tuning entails training models for additional epochs on more data, allowing customization of the model's behavior, tone, or output format.
- Used to make models act in specific ways, such as speaking like a lawyer or adopting the tone of Harry Potter.
- Unlike RAG, it focuses more on the form and tone of the output rather than knowledge augmentation.
🤔 Decision Dilemma: RAG or Fine Tuning?
- Emmanuel highlights the misconception that fine tuning injects new knowledge, emphasizing its role in shaping the output according to user needs.
- RAG is preferred for factual answers, as it extracts information directly from a specified context, ensuring higher accuracy.
- Fine tuning, on the other hand, is more about customizing the form and tone of the output.
🔄 The Verdict: A Balanced Approach?
- It's not a one-size-fits-all decision. The choice between RAG and fine tuning depends on specific use cases.
- Evaluating the decision involves understanding the goals: knowledge augmentation (RAG) or customization of form and tone (Fine Tuning).
- Perhaps a balanced approach, leveraging both techniques based on the desired outcomes.