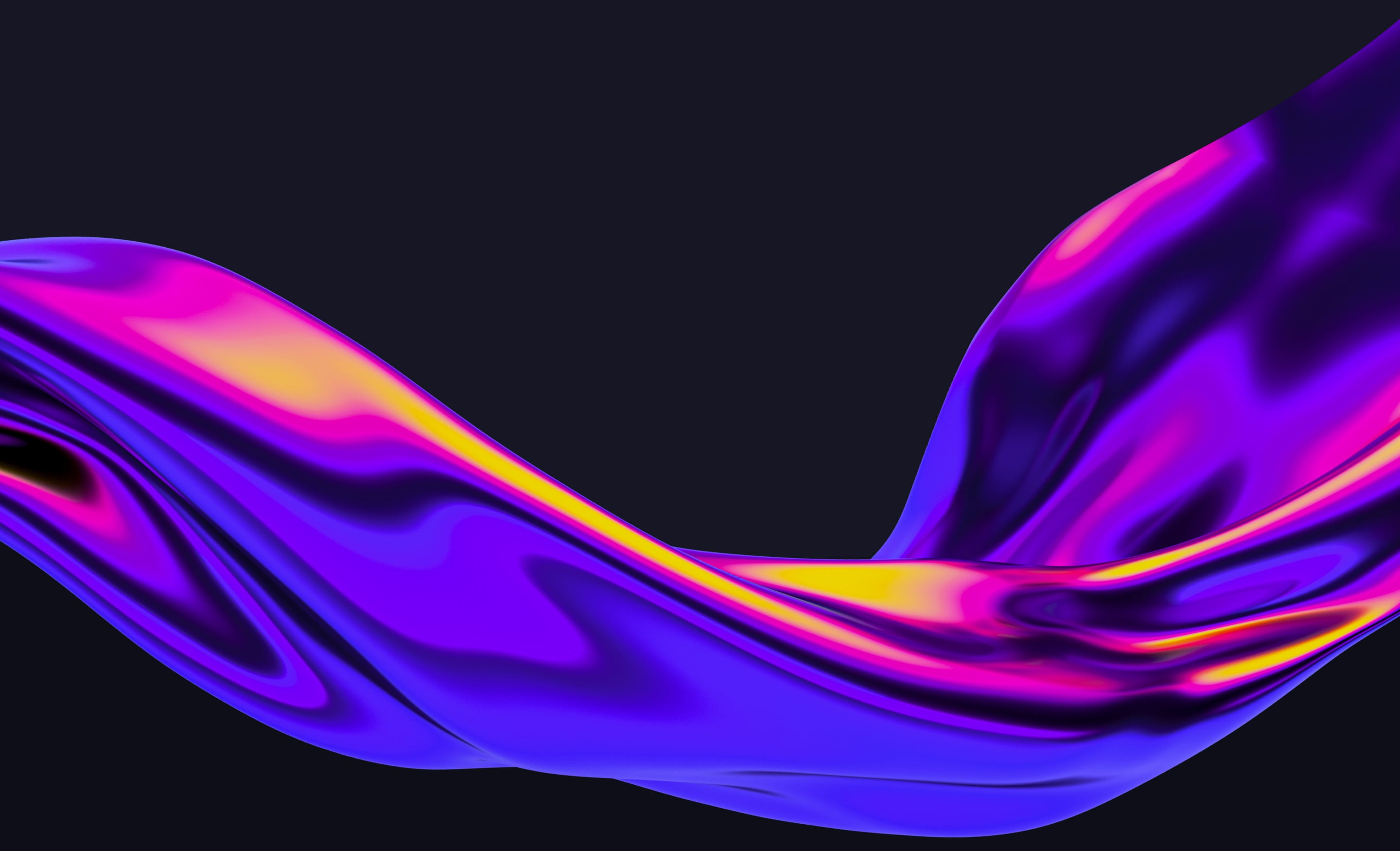
Hand labeling, a fundamental part of human-mediated machine intelligence, in today's age is akin to scribes hand-copying books post-Gutenberg. What's more is that the process is naive, dangerous, and expensive in light of the ever-growing world of alternatives which includes semi-supervised learning, weak supervision, and active learning.
The significant issues with hand labeling include the introduction of bias (and hand labels are neither interpretable nor explainable), the prohibitive costs (both financial costs and the time of subject matter experts), and the fact that there is no such thing as gold labels (even the most well-known hand labeled datasets have label error rates of at least 5%!).
We will explore the ways hand labeling has been negatively impacting ML solutions in production today, navigate the world of alternatives, and provide a framework for how to think about when to turn towards automation or manual annotation.